Next: Introduction to the functionalities
Up: Background on stability based
Previous: Stability indices
Statistical tests to assess the significance of clustering solutions
Consider a set of k-clusterings
, where
is a set of numbers of clusters and the
corresponding set of integrals computed according to eq. 2. Then we obtain a set of values
.
We can sort G obtaining
with values
in ascending order.
For each k-clustering we consider two groups of pairwise clustering similarities values separated by a threshold
(a reasonable threshold could be
.).
Thus we may obtain:
,
where
is computed according to eq. 1. If
represents the number of trials for estimating the
value of
then
is the number of times for which the similarity values are larger than
.
The
may be interpreted as the successes from
binomial populations with
parameters
. If the number of trials
is sufficiently large, and setting
as a random variable that counts
how many times
, we have that the following random variable, for sufficiently large values of
is distributed according
to a normal distribution:
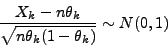 |
(5) |
A sum of i.i.d. squared normal variables is distributed according to a
distribution:
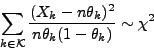 |
(6) |
Considering the null hypothesis
: all the
are equal to
, where the unknown
is
estimated through its pooled estimate
,
then the null hypothesis may be evaluated against the alternative hypothesis that the
are not all equal
using the statistic
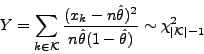 |
(7) |
If
we may reject the null hypothesis at
significance level, that
is we may conclude that with probability
the considered proportions are different, and hence that at least
one k-clustering significantly differ from the others.
Using the above test we start considering all the k-clustering. If a significant
difference is registered according to the statistical test we exclude the last clustering
(according to the sorting of
). This is repeated until no significant difference is detected (or until only 1 clustering is left off): the set of
the remaining (top sorted) k-clusterings represents the set of the estimate stable number of clusters discovered (at
significance level).
It is worth noting that the above
-based procedure be also applied to automatically find the optimal number
of clusters independently of the applied perturbation method.
Anyway, note that with the previous
-based statistical test we implicitly assume that some probability distributions are normal.
Using the classical Bernstein inequality [12] we may apply a partially "distribution independent" approach to assess the significance of the discovered clustering.
In this way we can apply a statistical test without any assumption about the distribution of the random variables. For more details see [5],
downloadable from:
http://homes.dsi.unimi.it/valenti/papers/bertoni-vale-cisi06.pdf.
Next: Introduction to the functionalities
Up: Background on stability based
Previous: Stability indices